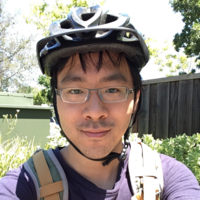
I am an applied scientist at Pinterest working on GPUs, ranking models, and ML system. Before that, I got my PhD from the Machine Learning Department at Carnegie Mellon University, working on semidefinite solvers and differentiable optimization layers with Zico Kolter. Even before that, I got my BS from the CSIE Department at National Taiwan University, working with Prof. Chih-Jen Lin on optimization of SVMs and its convergence properties.
Projects
Differentiable satisfiability solver as a layer [12]
- Logical reasoning within deep learning using a differentiable SAT solver.
- Approximates MAXSAT with Goemans-Williamson SDP, and differentiates through the low-rank SDP.
- Wrap-level GPU optimization with CUDA C. Best paper honorable mention at ICML ’19.
Low-rank semidefinite solvers for MAXCUT and MAXSAT [8] [11]
- The first proof on the convergence to global optimum for a low-rank SDP method using the stable-manifold theorem.
- Breakthrough in scaling semidefinite program to millions of variables.
- Orders of magnitude faster than other existing methods in experiments.
The distributed common-direction solver for linear classification [6] [10]
- Reuse gradient information to reduce communication time in distributed optimization.
- Converges linearly in optimal rate and enjoys local quadratic convergence.
- Outperforms the state-of-the-art first- and second-order methods in experiments.
Global linear convergence for non-strongly convex problems [2]
- The first global linear convergence rate for first-order methods on non-strongly convex problems.
- Applied to cyclic coordinate descent methods for dual SVC and SVR, published in JMLR.
- Solves the open problem of convergence rate of the Gauss-Seidel method on PSD matrices.
Education
- PhD student at MLD, Carnegie Mellon University, Aug 2015--Now
- B.S., CSIE, National Taiwan University, Sep 2008--Jun 2012.
Publications
-
Community detection using fast low-cardinality semidefinite programming
[code]
Po-Wei Wang and J. Zico Kolter. NeurIPS, 2020 -
Efficient semidefinite-programming-based inference for binary and multi-class MRFs
[code]
Chirag Pabbaraju, Po-Wei Wang, and J. Zico Kolter. NeurIPS, 2020 -
Differentiable learning of numerical rules in knowledge graphs
[slide]
Po-Wei Wang, Daria Stepanova, Csaba Domokos, J. Zico Kolter. ICLR, 2020 -
SATNet: Bridging deep learning and logical reasoning using a differentiable satisfiability solver
[code] [slide] [poster] [Sudoku dataset, parity dataset]
Po-Wei Wang, Priya L. Donti, Bryan Wilder, Zico Kolter. ICML, 2019 (Best paper Honorable mention) -
Low-rank semidefinite programming for the MAX2SAT problem
[code] [slide]
Po-Wei Wang, J. Zico Kolter. AAAI, 2019 -
The Common-directions Method for Regularized Empirical Risk Minimization
[supp and exp code] [slide]
Po-Wei Wang, Ching-pei Lee, and Chih-Jen Lin. JMLR, 2019. -
Realtime query completion via deep language models
[code]
Po-Wei Wang, Huan Zhang, Vijai Mohan, Inderjit S. Dhillon, and J. Zico Kolter. SIGIR Workshop on eCommerce, 2018 -
The Mixing method: low-rank coordinate descent for semidefinite programming with diagonal constraints
[code] [poster]
Po-Wei Wang, Wei-Cheng Chang, and J. Zico Kolter. arXiv preprint, 2017. -
Polynomial optimization methods for matrix factorization
Po-Wei Wang, Chun-Liang Li, and J. Zico Kolter. AAAI, 2017. -
Limited-memory common-directions method for distributed optimization and its application on empirical risk minimization
[supp and exp code]
[Implemented in Distributed LIBLINEAR]
Ching-pei Lee, Po-Wei Wang, Weizhu Chen, and Chih-Jen Lin. SDM, 2017. -
The Mixing method for Maxcut-SDP problem
Po-Wei Wang and J. Zico Kolter. NIPS LHDS Workshop, 2016. -
Epigraph projections for fast general convex programming
[github]
[slide]
Po-Wei Wang, Matt Wytock, and J. Zico Kolter. ICML, 2016. -
Convex programming with fast proximal and linear operators
[github]
Matt Wytock, Po-Wei Wang and J. Zico Kolter. NIPS Optimization Workshop, 2015. -
Iteration Complexity of Feasible Descent Methods
for Convex Optimization
[slide]
Po-Wei Wang and Chih-Jen Lin. JMLR, 15(2014), 1523-1548. -
Support Vector Machines
in
Data Classification: Algorithms and Applications.
Po-Wei Wang and Chih-Jen Lin. CRC Press, 2014.
Links
-
Backup Site for Paul Tseng
- The old website was removed by UW, and this backup site is dedicated to him. It's recovered from WayBackMachine. Another more complete backup could be found here.
Honors
- Best paper honorable mention from ICML 2019.
- Scholarship from Amazon A9.com for works on deep query completion.
- Our team NTU RobotPal earned the 3rd place in Robocup Standard Platform League.
- Top 16, 2010 Singapore, photos
- 3rd place, 2011 Istanbul, photos and videos
- Our team are Champions for both tracks in the Yahoo KDD Cup 2011 on Music Rating Prediction.
- NTU Presidential Awards (top 5%).
- Undergraduate scholarship from Macronix Education Foundation.
- Grant and prize from the National Science Council for Creative Undergraduate Research.